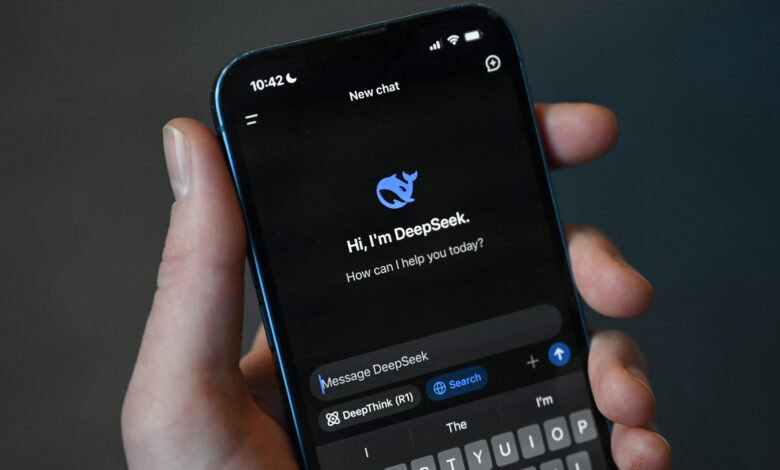
At MyDailyTech.net , we’re always on the lookout for groundbreaking advancements in technology, and the story of DeepSeek is nothing short of remarkable. This Chinese startup has made waves by building one of the world’s most powerful artificial intelligence (AI) systems at a fraction of the cost typically required. In this article, we’ll explore how DeepSeek achieved this feat using innovative techniques like the “mixture of experts” method and advanced computational strategies.
The Rise of DeepSeek: Building AI for Less
Last month, financial markets took notice when DeepSeek announced its latest AI model, which rivals some of the best systems from global giants like Meta and OpenAI—but with significantly lower resource requirements. While traditional AI companies use supercomputers equipped with 16,000 specialized chips or more to train their models, DeepSeek managed to achieve similar results with just 2,000 chips . Their total expenditure? A mere $6 million , compared to the tens or hundreds of millions spent by competitors.
This achievement didn’t happen overnight. In a research paper published shortly after Christmas, DeepSeek detailed the technological innovations that allowed them to slash costs while maintaining high performance. Let’s break down their approach.
Understanding Neural Networks: The Backbone of Modern AI
Before diving into DeepSeek’s methods, it’s important to understand how AI technologies are built. Most cutting-edge AI systems rely on neural networks , mathematical structures inspired by the human brain. These networks learn skills by analyzing vast amounts of data, such as text, images, audio, and video.
Training these neural networks requires enormous computing power because they must process terabytes—or even petabytes—of information. For example:
- Meta’s Llama series consumes billions of dollars worth of resources.
- OpenAI’s GPT models require months of continuous computation across thousands of GPUs.
The primary tools powering these efforts are graphics processing units (GPUs) , originally designed for rendering graphics in video games. Companies like NVIDIA dominate the market with their high-performance GPUs, but these come with hefty price tags—upward of $40,000 per chip—and consume massive amounts of electricity.
DeepSeek’s Secret Sauce: Mixture of Experts Method
So, how did DeepSeek reduce costs so dramatically? One key innovation lies in the mixture of experts method, an approach that rethinks the way neural networks are structured.
Traditional Approach vs. Mixture of Experts
Traditionally, companies build a single, monolithic neural network capable of learning all patterns across diverse datasets. However, this setup demands constant communication between GPU chips, leading to inefficiencies and higher costs.
DeepSeek tackled this issue by dividing the system into multiple smaller neural networks, each specializing in a specific domain:
- One network focuses on poetry.
- Another handles computer programming.
- Yet another specializes in biology, physics, or other fields.
These smaller “experts” can concentrate on their respective areas without needing constant interaction with others. To ensure cohesion, DeepSeek introduced a generalist system that acts as a coordinator, enabling limited communication between experts where necessary. Think of it as an editor overseeing a team of specialist reporters.
This modular architecture drastically reduces the need for inter-chip communication, saving both time and energy.
Cutting Costs Through Mathematical Efficiency
Beyond structural changes, DeepSeek employed clever mathematical tricks to further optimize performance. Here’s how:
Reducing Precision Without Sacrificing Accuracy
Neural networks perform countless multiplications during training. Typically, these calculations involve numbers stored in 16-bit memory . DeepSeek reduced this to 8 bits , effectively halving the storage requirement.
While this reduction sacrifices some precision, it turns out that neural networks don’t require absolute accuracy at every step. By tolerating slight errors early in the process, DeepSeek achieved comparable results without compromising overall quality.
Balancing Precision During Final Calculations
To strike a balance, DeepSeek retained higher precision during critical stages of computation. Specifically, they expanded the final results of multiplication operations to 32 bits , ensuring greater accuracy where it mattered most. This hybrid approach maximizes efficiency without sacrificing essential accuracy.
Why Didn’t Others Do This Before?
If these techniques seem straightforward, why haven’t other major players adopted them already? There are several reasons:
- Risk and Experimentation : Developing novel approaches requires significant upfront investment, often resulting in failure. Many organizations shy away from taking such risks.
- Proprietary Secrets : Some companies may already be employing similar strategies but choose not to disclose them publicly.
- Talent Gap : Writing efficient code for GPUs demands specialized expertise, which only top-tier labs possess.
As Tim Dettmers, a researcher at the Allen Institute for Artificial Intelligence, explained, “You have to put a lot of money on the line to try new things—and often, they fail.” DeepSeek embraced this risk and succeeded, paving the way for future innovations.
Implications for the Future of AI
DeepSeek’s breakthrough could democratize access to advanced AI technologies. By reducing costs and improving efficiency, smaller startups and research institutions can now compete with tech giants. Additionally, sharing their methods openly encourages collaboration and accelerates progress across the industry.
For businesses looking to integrate AI solutions, DeepSeek’s innovations offer hope for scalable, cost-effective implementations. Whether you’re automating workflows, enhancing customer service, or developing predictive analytics, these advancements make AI more accessible than ever.